Reduced-order hybrid models for real-time optimisation and control of additive manufacturing processes
The thesis, funded by the project, aims to develop a new methodology for the design and control of the Additive Manufacturing (AM) process of components with complex geometries and optimised topologies, implemented in AIMEN. It involves the development of a software tool that can guarantee, in real time, the manufacturability, reliability and quality of the target component from its initial design.
To achieve this, the hybridization of numerical simulation based on physical models with the data obtained during manufacturing (testing, prototyping and final manufacturing) is used. Once the calibration of the models associated with the thermal behaviour of the manufactured part has been conducted through the data, an attempt will be made to obtain hybrid models of reduced order to give an agile and reliable response to the complete model. This will allow the models to respond in real time, allowing design optimisation, automated process planning, and the definition of new online control and inspection strategies.
In conjunction with these general objectives, the following technical objectives are defined:
- To define the mathematical models associated with the direct problems at the macro scales of the Additive Manufacturing (AM) process by Direct Energy Deposition (DED).
- To define: a) the test-tube configurations; b) the critical process variables; c) the physical parameters of the feedstock and base materials before, during and after deposition; d) the batteries of experiments; and e) the characterisation tests that will provide the necessary information to adequately calibrate the direct models.
- Identify and deploy the necessary sensors in the production setup to reliably acquire the process information required for the calibration of the models.
- Develop hybrid reduced order models and apply them in mesoscale and macroscopic models for FA DED processes.
- To calibrate and validate the developed reduced order methodology in different manufacturing scenarios.
Project details
Reference: 12_IN606D_2021_2606987
Acronym:
Period: 29/12/2021- 28/12/2024
Principal Investigators:
P. Quintela.
Research Team:
P. Barral
P. Quintela, J. Rodríguez
M. Picos (Ph.D. Student AIMEN)
M. Salgueiro (AIMEN)
Funding: Axudas para a formación de doutores e doutoras das universidades do Sistema universitario de Galicia (SUG) en empresas, Programa de doutoramentos industriais, Xunta de Galicia.
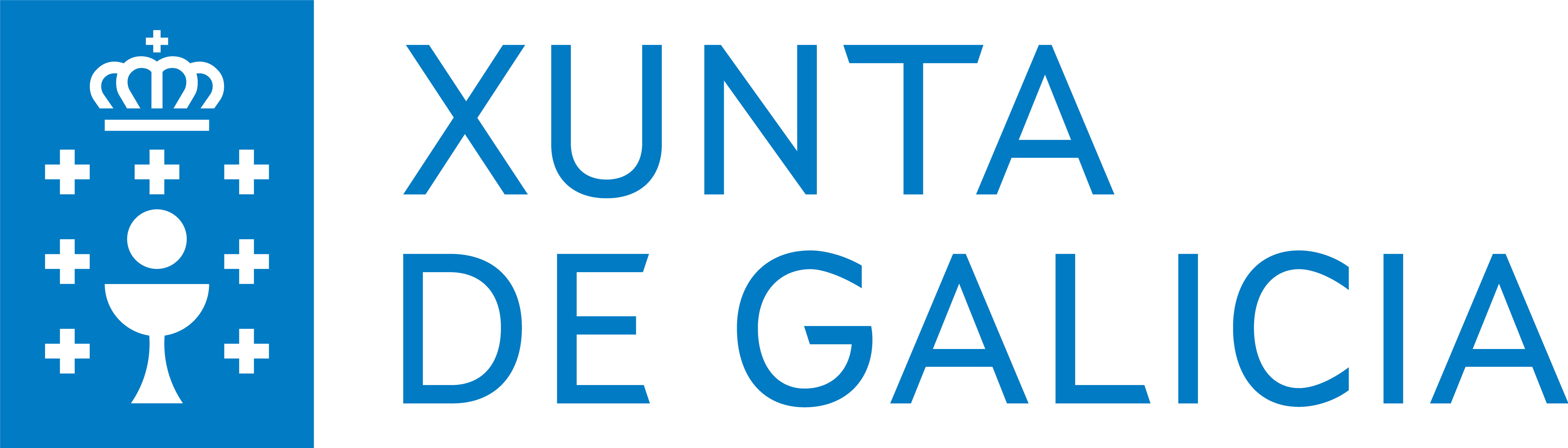